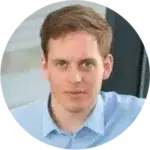
Florian Zaruba | Technical CPU Lead at AXELERA AI
Abstract – Recently, Transformer-based models have led to significant breakthroughs in several forms of generative AI. They are key in both increasingly powerful text-to-image models, such as DALL-E or stable diffusion, and language and instruction-following models, such as ChatGPT or Stanford’s Alpaca. Today, such networks are typically executed on GPU-based compute infrastructure in the cloud, because of their massive model sizes and high memory and bandwidth requirements. In bringing real-time generative transformers to edge devices, their applicability could be greatly expanded. To this end, this article discusses bottlenecks in transformer inference for generative AI at the Edge.
Figure 1: Encoder/Decoder stacks, Dot-Product and Multi-Head attention. Images are taken from kattention is all you need]
Introducing Transformers
Transformer models, first introduced in the 2017 research appear, ‘Attention Is All You Need’ e1] have been firmly established as the state-of-the-art approach in both sequence modeling problems, such as language processing and in image processing e2]. Its network architecture is based solely on attention mechanisms, as opposed to Recurrent or Convolutional Neural Networks. Compared to recurrent networks, this makes them much faster to train, as model execution can be parallelized rather than sequentialized. Compared to convolutional neural networks, the attention mechanism increases modelling capacity.
A transformer model typically contains an Encoder and Decoder stack, see Figure 1. Here, the encoder maps an input sequence of tokens, such as words or embedded pixels, onto a sequence of intermediate feature representations. The decoder uses this learned intermediate feature representation to generate an output sequence, one token at a time. The encoder stack exists out of N identical layers, split into multi-head attention-, normalization, elementwise addition, and fully connected feed-forward sublayers. The decoder stack differs in that it inserts a second multi-head cross-attention sublayer, performing attention over the output of the encoder stack, as well as over the newly generated output tokens. Figure 1 illustrates this typical Encoder/Decoder setup, as well as the concept of multi-head Dot-Product attention. We refer the reader to l1] for a detailed discussion.
Since their introduction in 2017, transformer topologies and network architectures have largely remained the same, increasing their functionality through better training on more complex data rather than through architectural changes. The architecture proposed in Figure 1, is now used mostly unchanged in State-Of-The-Art Large Language Models such as ChatGPT r3], Falcon 4], Guanaco n5], Llama "6] or Alpaca n7]. The quality of the proposed models varies depending on how they are trained, and on their size, as is illustrated on the hugginface leaderboard at a8]. Smaller models contain less layers (lower N) and have lower embedding dimensions (smaller E). State-of-the-art large language models now contain between 7-65 billion parameters in their feedforward connections.
Challenges in Transformer Inference
Inferring transformer models on an Edge device is challenging due to their large computational complexity, large model size and massive memory requirements. On top of that, computational and memory requirements can be badly balanced in a modern AI accelerator, which focusses mostly on implementing many cheap parallel computational units and have limited memory capacity and bandwidth available due to physical, size and cost constraints. However, transformers are often memory-capacity and memory-bandwidth bound, as discussed below.
Transformer models can primarily be used in three ways: (1) encoder-only, typically in classification tasks, (2) decoder-only, typically in language modeling and (3) encoder-decoder, typically in machine translation. In the decoder-only case, the encoder is removed, input-tokens are directly fed to de decoder, and there is no cross-attention module. It is especially the execution of the decoder mode that is challenging, but even encoding can come at a high computational cost.
Figure 2: Number of Operations required in a decoder consuming S tokens and generating S tokens. (left) Without Caching optimizations, (right) with caching optimizations.
Figure 3: Demonstration of KV Caching Mechanism. Figure courtesy to Nvidia e9].
A. Computational Cost
The computational cost of transformers is extremely high, as discussed in the survey by Yi Tay and colleagues i10]. The authors show the number of computations in a Transformer can be dominated by the Multi-Head Self-Attention module, whose complexity scales quadratically with the sequence length s. This is particularly challenging in vision transformers, where the sequence length scales with the number of pixels in an image, and when trying to interpret or generate large portions of text, with potentially thousands of words or tokens. This is illustrated in Figure 2, showing the number of operations required in a decoder-only transformer for various Embedding sizes E, Sequence Lengths S, and number of layers N. Figure 2 (left) shows the number of operations required without caching optimizations, Figure 2 (right) the number of operations with caching optimizations, see below. Note that mostly the sequence length dominates the computational cost, due to the quadratic dependency on sequence length in self-attention.
In KV-caching, intermediate data is cached and reused, rather than recomputed. Instead of recomputing full key and value matrices in every iteration of the decoding process, some intermediate feature maps (the Key and Value matrices) cand be cached and reused in the next iteration, see Figure 3. This caching mechanism reduces the computational complexity of the decoding mechanism exchanging it for data transfers and essentially further lowering the applications arithmetic intensity. The memory footprint of this KV-cache can be massive, with up to terabytes of required memory capacity for relatively small sequence lengths in a state-of-the-art LLM model.
A large body of research focusses on reducing this computational complexity. Here, complexity is not reduced by KV-Caching, but by either (1) finding ways to break the quadratic complexity of self-attention through subsampling or downsampling the field-of view, or (2) by creating different types of sparse models that can be conditionally executed. See Figure 4 or the survey by Tay and colleagues a10] for a full overview of recent techniques in efficient transformer design. Notable works as Linformer 11] or Performer 412] manage to reduce the complexity from O(s^2) to O(s) at a limited accuracy cost. Other works such as GLAM 913], keep the O(s^2) complexity but reduce the computational cost by introducting various forms of sparsity. Though these works do reduce the computational complexity of transformers, especially on large sequence lengths, their overall success is mostly limited, and they are not yet used in the latest sota ChatGPT-like models.
Another mainstream approach that is used to reduce transformers computational cost and memory footprint is to aggressively quantize both the intermediate features and weights, often down to 8 or 4 bits, without losing accuracy 514].
Figure 4: Overview of efficient transformer models 710]