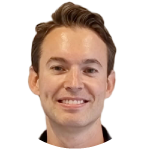
Bram Verhoef | Director of Customer Engineering & Success at AXELERA AI
To create a high-performing and highly energy efficient AI processing unit (AIPU) that obsoletes extensive model retraining, our engineers took a radically different approach to data processing. Through unique quantization methods and a proprietary system architecture, Axelera is able to offer the most powerful AI accelerator for the edge you can buy today. In this blog, you can read all about our unique quantization techniques.
Industry-leading performance and usability
Our Metis acceleration hardware leads the industry, because of our unique combination of advanced technologies. This is how our sophisticated quantization flow methodology enables Metis’ high performance and efficiency.
- Metis is very user-friendly, not in the least because of the quantization techniques that are applied. Axelera AI uses Post-Training-Quantization (PTQ) techniques. These quantization techniques do not require the user to perform any retraining of the model, which would be time-, compute- and cost-intensive. Instead, PTQ can be performed quickly, automatically, and with very little data.
- Metis is also fast, energy-efficient and cost-effective. This is the result of innovative hardware design, like digital in-memory-computation and RISC-V, but also from the efficiency of the algorithms running on it. Our efficient digital in-memory-computation works hand in hand with quantization of the AI algorithms. The quantization process casts the numerical format of the AI algorithm elements into a more efficient format, compatible with Metis. For this, Axelera AI has developed an accurate, fast and easy-to-use quantization technique.
Model | Deviation from FP32 accuracy |
---|---|
ResNet-34 | -0.1% |
ResNet-50v1.5 | -0.1% |
SSD-MobileNetV1 | -0.3% |
YoloV5s-ReLu | -0.9% |
Accuracy drop @ INT8
Highly accurate quantization technique
In combination with the mixed–precision arithmetic of the Axelera Metis AIPU, our AI accelerators can deliver an accuracy practically indistinguishable from a reference 32-bit floating point model. As an example, Metis AIPU can run the ResNet50v1.5 neural network processing, at a full processing speed of 3,200 frames per second, with a relative accuracy of 99.9%.
Technical details of our post-training quantization method
To reach high performance, AI accelerators often deploy 8-bit integer processing of the most compute-intensive parts of neural network calculations instead of using 32-bit floating-point arithmetic. To do so, a quantization of the data from 32-bit to 8-bit needs to be done.
The Post-Training Quantization (PTQ) technique begins with the user providing around hundred images. These images are processed through the full-precision model while detailed statistics are collected. Once this process is complete, the gathered statistics are used to compute quantization parameters, which are then applied to quantize the weights and activations to INT8 and other precisions in both hardware and software.
Additionally, the quantization technique modifies the compute graph to enhance quantization accuracy. This may involve operator folding and fusion, as well as reordering graph nodes.
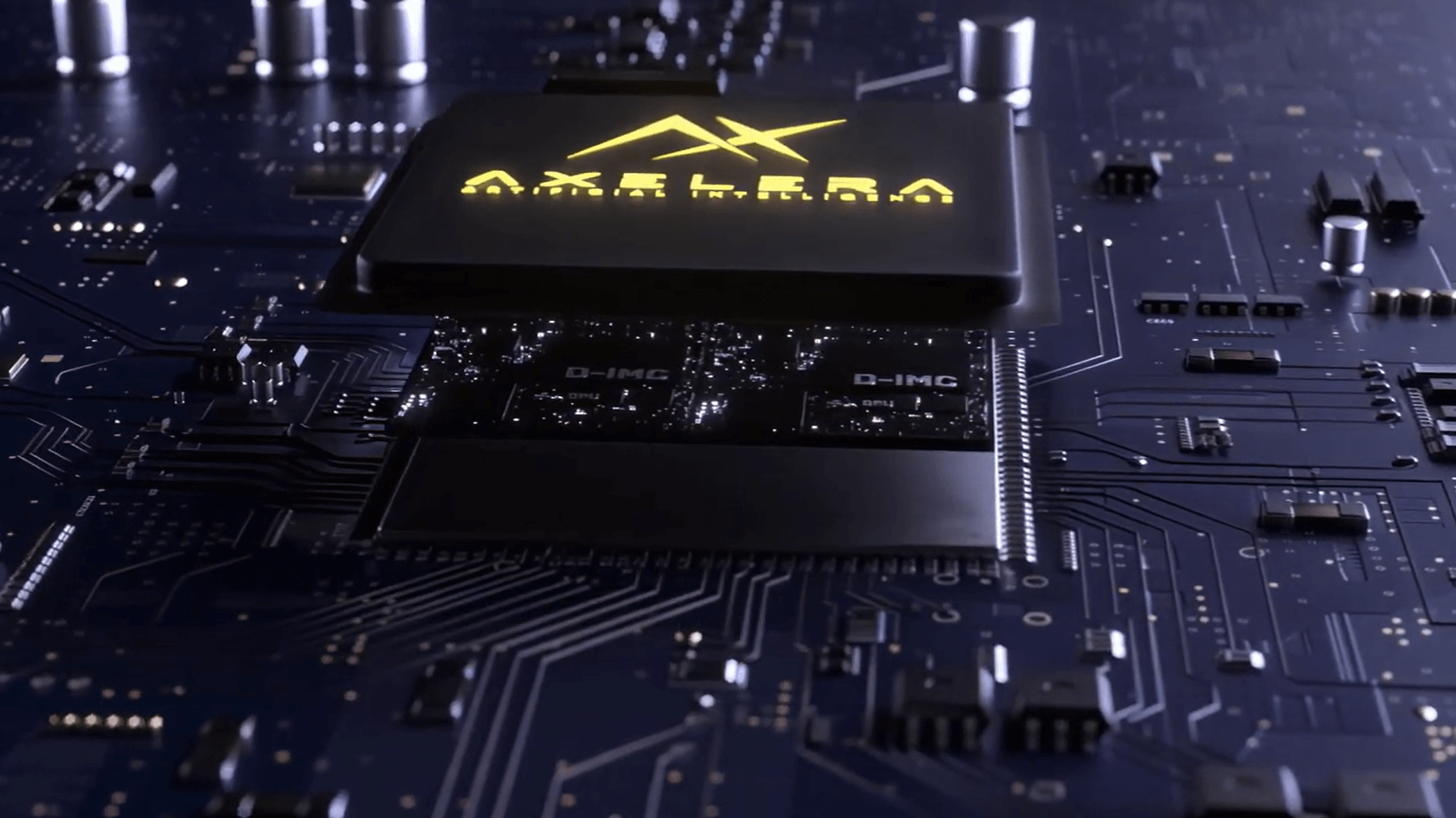
Our radically different approach to data processing
From the outset, we designed our quantization method with two primary goals in mind. The first goal is achieving high efficiency, the second is high accuracy. Our quantized models typically maintain accuracy comparable to full-precision models.
To ensure this high accuracy, we begin with a comprehensive understanding of our hardware, as the quantization techniques employed depend on the specific hardware in use. Additionally, we utilize various statistical and graph optimization techniques, many of which were developed in-house.
Compatible with Various Neural Networks
By employing a generic quantization flow methodology, our systems can be applied to a wide variety of neural networks while minimizing accuracy loss.
Our quantization scheme and hardware allow developers to efficiently deploy an extremely wide variety of operators. This means that Axelera AI's hardware and quantization methods can support many different types of neural network architectures and applications.
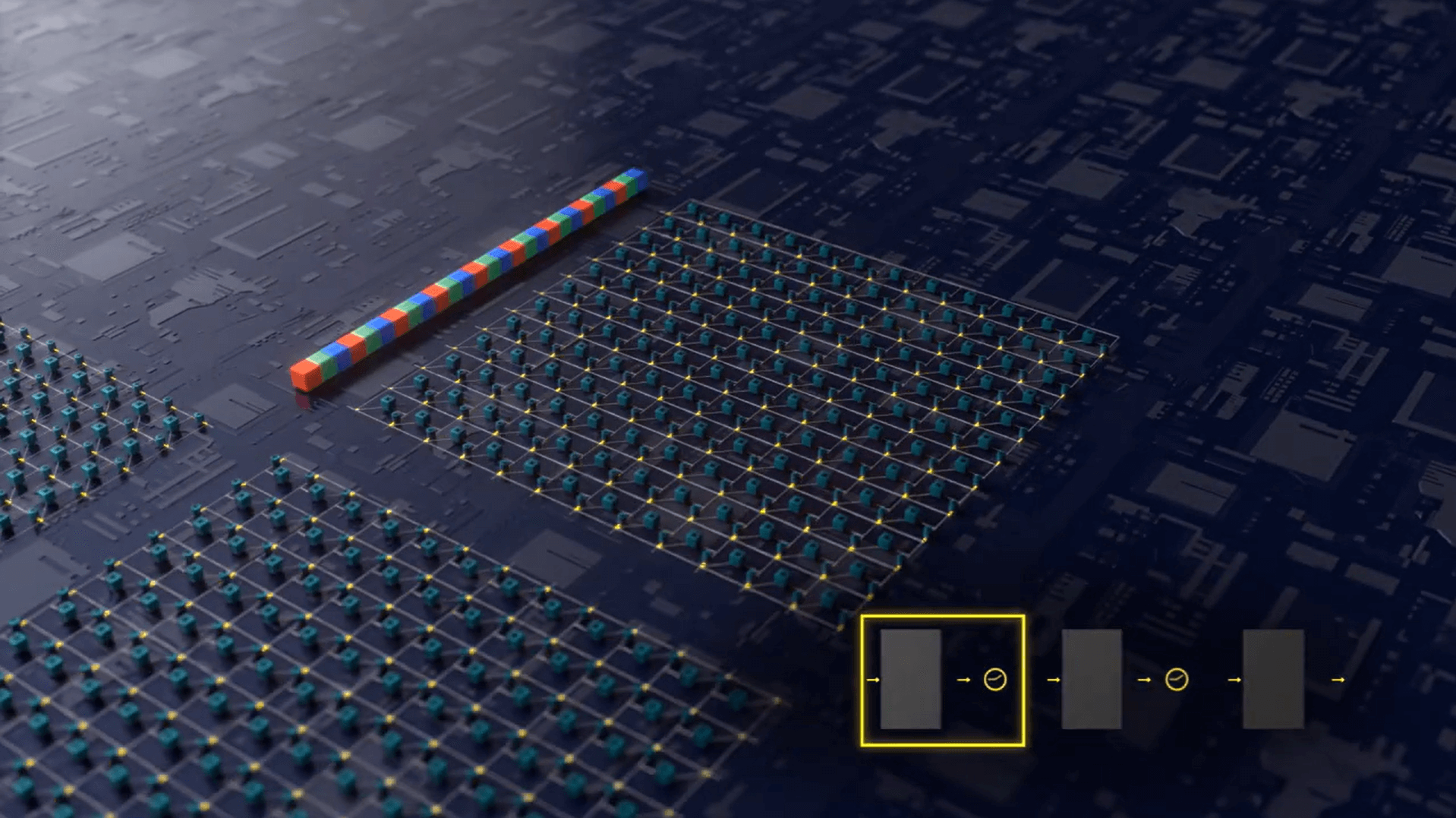