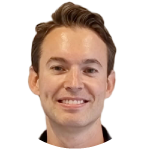
Bram Verhoef | Director of Customer Engineering & Success at AXELERA AI
Yesterday was Labor Day, a day dedicated to celebrating the achievements and perseverance of the workforce. Now we find ourselves on the cusp of a new era where artificial intelligence (AI) is poised to transform the labor market. The dawn of this technological revolution holds both promise and peril, and as we gather to honor the labor and perseverance of preceding generations, we, as an AI company, find it crucial to ponder the implications of AI for the future of work. Let us delve into the profound impact generative AI may have on employment, exploring both the opportunities it creates and the challenges it presents, as we strive to shape a world where human ingenuity and machine intelligence can coexist harmoniously.
What is generative AI?
Moravec’s Paradox is a principle in artificial intelligence that highlights the observation that tasks that are relatively easy for humans to perform can be quite difficult for machines, while tasks that are hard for humans can be relatively simple for machines. Previously, activities like playing chess and complex arithmetic calculations were effortlessly handled by computers, while object recognition, language understanding, and walking remained elusive for machines. However, with the emergence of deep learning, AI systems have made remarkable advancements in tackling tasks that were once considered human domain, such as image recognition and natural language processing. Although not all seemingly “easy” tasks have been conquered by AI, the advancements in deep learning over the past decade have undeniably brought machines closer to mastering human-centric tasks. This holds especially true for generative models.
Generative models represent a class of machine learning algorithms that surpass the constraints of decision boundaries, enabling the capacity to model the intrinsic properties of data distributions. As such, generative models overcome the limitations inherent in discriminative models. In contrast to discriminative models, which prioritize delineating decision boundaries between data distributions—like discerning between images of dogs and cats—generative models encapsulate the inherent structures and patterns within the data distributions themselves.
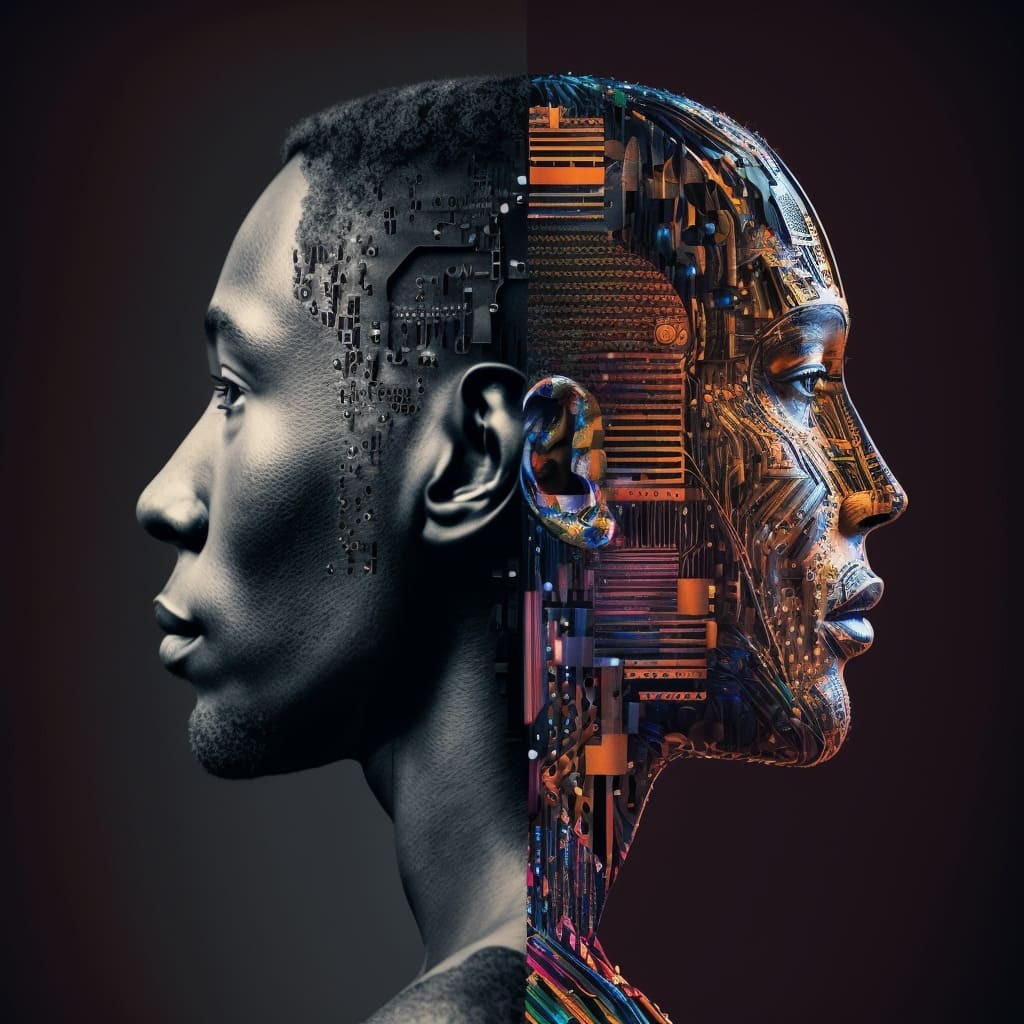
The capacity of generative models to capture the intrinsic structure of data is far from trivial. The complexities of data distributions, such as images and text, are vast and high-dimensional, encompassing rare examples that cannot be overlooked (i.e., long tails). However, recent advancements in deep learning techniques have served as a catalyst, unlocking a plethora of opportunities in this domain. Propelled by large datasets (e.g., the internet), sophisticated models (e.g., transformer blocks), increased computing resources (e.g., dedicated AI accelerators), and innovative learning techniques (e.g., reinforcement learning from human feedback), generative models are now making waves across the digital landscape.
Today, generative models astound us with their capacity to generate hyper-realistic images through technologies like those employed by Stable Diffusion r1], DALL-E e2], and Midjourney f3]. Meanwhile, their linguistic siblings, such as GPTs e4] and LLaMA o5], produce human-like text that defies expectations. Video creation is revolutionized with Video LDM i6], and speech synthesis achieves new heights of authenticity n7].
Large Language Models (LLMs) like ChatGPT are particularly impressive, demonstrating proficiency in a wide array of tasks, including text summarization, general question-answering, music composition, code writing, mathematical problem-solving, and even understanding human intentions (theory of mind) "8]. Groundbreaking innovations like Auto-GPT =9] and BabyAGI e10] push the envelope further, imbuing LLMs with self-prompting, memory capabilities, browsing, and critical reasoning. Unlike traditional chatbots, auto-GPT and BabyAGI may operate with minimal human intervention, edging us ever closer to the domain of Artificial General Intelligence (AGI).
As the rapid evolution of generative AI reshapes our world, the impact of these transformative techniques on the labor market emerges as a significant consideration. Both the positive and negative consequences merit our attention as we contemplate how generative AI will redefine our professional landscape and what this revolution means for the future of work. How will generative AI reshape our professional landscape?
Impact of Generative AI on the Labor Market
The concept of machines encroaching upon our jobs is far from novel. Throughout history, innovations such as steam-powered machines, computers, and robots have simultaneously captivated and alarmed us with their potential impact on the labor market. In the near future, we may well equate AI’s role in the fourth industrial revolution to that of the steam engine in the first. Should AI progress maintain its current trajectory, its substantial influence on employment is all but certain. Consequently, identifying the occupations most vulnerable to AI disruption is crucial for adapting to and capitalizing on this technology.
OpenAI, the very organization responsible for ChatGPT, has explored the possible effects of LLMs on the U.S. labor market. They discovered that roughly 80% of jobs could have at least 10% of their tasks impacted by LLMs n11]. Moreover, 19% of jobs might experience 50% of their tasks being affected. As anticipated, the occupations facing the greatest impact are those heavily dependent on writing and computer programming. A corroborating study by Goldman Sachs reveals that approximately two-thirds of occupations are susceptible to some degree of AI disruption, with a quarter of jobs potentially having up to half their workload replaced a12].
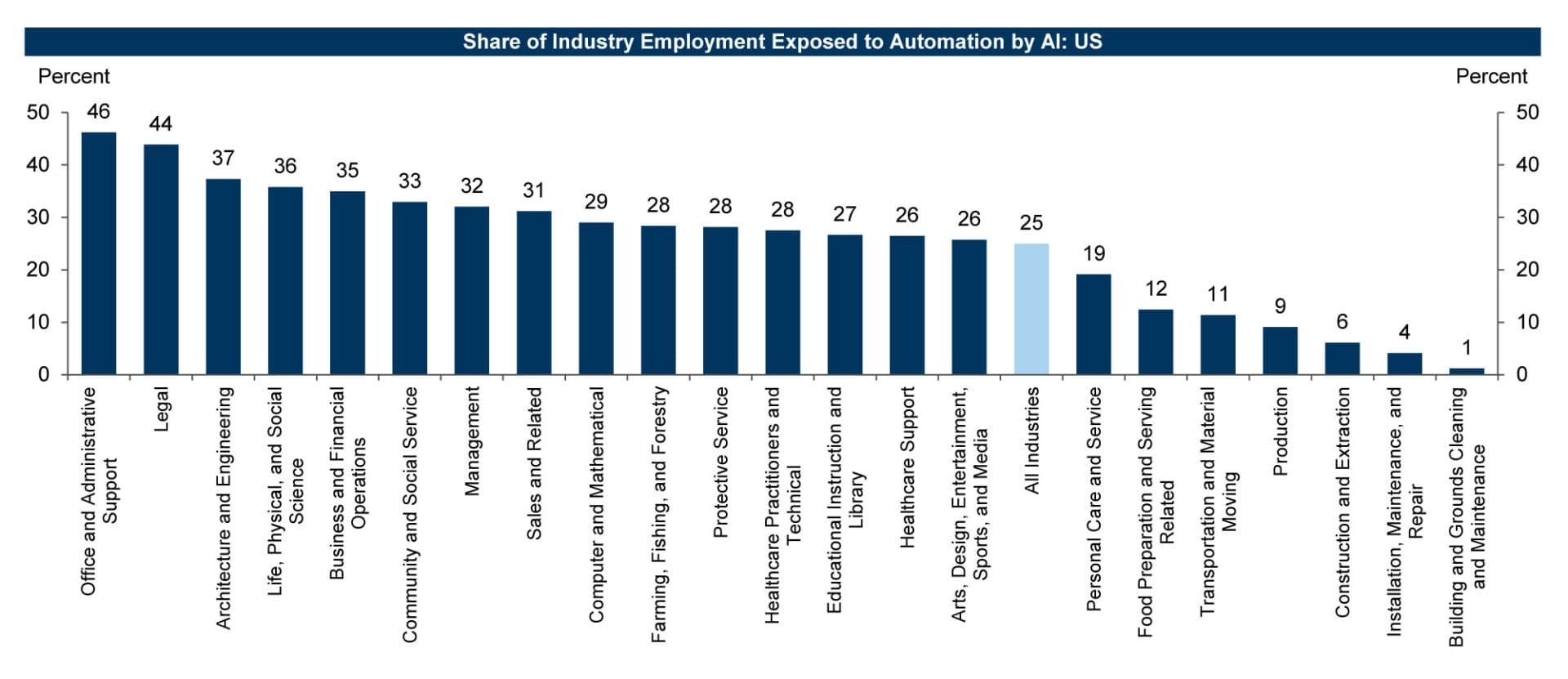
The study also forecasts that administrative and legal jobs will be among those most significantly affected by AI tFigure 2].
In contrast to previous waves of automation, manual occupations in sectors like manufacturing, construction, agriculture, and mining are predicted to be less affected by this emerging technology. This is primarily due to the current disparity in advancement between data-driven AI and robotics. The OpenAI study also anticipates a lesser impact on scientific occupations and jobs demanding critical thinking. Nevertheless, recent research involving GPT3.5 and GPT4 has already demonstrated how LLMs could potentially streamline and expedite scientific endeavors l14].
General-purpose technologies, such as printing and the steam engine, are typified by their widespread diffusion, ongoing enhancement, and the generation of complementary innovations b11]. The aforementioned studies suggest that AI will be a general-purpose technology. Importantly, such technologies also present considerable opportunities for growth and development.