What happens when you take one of the most demanding computer vision models, push it to 8K resolution, and run it live on the edge—right in the middle of the world’s biggest security tech trade show? At ISC West 2025, that’s exactly what we did. Here’s how.
Just a few months ago at CES I was speaking with a nationwide retailer in the process of upgrading its store camera systems to 4K. We discussed its AI strategy given that many state-of-the-art models are still trained at HD resolutions or less. Reasons for this are that training at higher resolutions is more computationally expensive, and labelling high-resolution datasets is resource intensive. Yet the point of inferencing at high resolution is often just to increase the distance from the camera at which objects can be detected, and not anything related to the model’s fundamental accuracy.
How We Cracked High-Resolution Inferencing at the Edge
With conventional inferencing, high-resolution video is downsized to the native model input resolution, producing a loss of information prior to inferencing. Tiling techniques such as SAHI mitigate this loss by subdividing each input image into a grid, running the model on each tile individually, and then reconstructing all detections with respect to their position in the original image.
A key Axelera AI differentiator is the ability to rapidly and efficiently scale up inferencing by using multiple cores and chips. So we decided to showcase the popular, yet computationally-demanding, YOLOv8l model running on Metis with an IP camera at 8K resolution. Developing this capability – not just for demo but as a general-purpose SDK feature that anyone can use with their own models – is technically quite challenging.
YOLOv8l is a large model with 43.7M parameters compared to the industry benchmark SSD-MobileNetv2, which has 3.4M parameters. It can take over 100 times longer to run, even before tiling. YOLOv8l’s native input size is 640x640, which subdivides an input video at 8K resolution (7680x4320) into a grid of 12x7 tiles, allowing for some vertical overlapping.
In addition, to ensure accurate detection of objects spanning multiple tiles, the original, downsized image is also used as a model input, for a total of 85 parallel streams. This processing must be split efficiently end-to-end between the host processor and Metis accelerator, with the host preparing vast amounts of camera data for inferencing Tasks include:
- Color conversion
- Scaling
- Letterboxing
- Tensor conversion
- Post-processing
The latter of these applies algorithms such as non-maximal suppression to the output, removing duplicate detections between tiles.
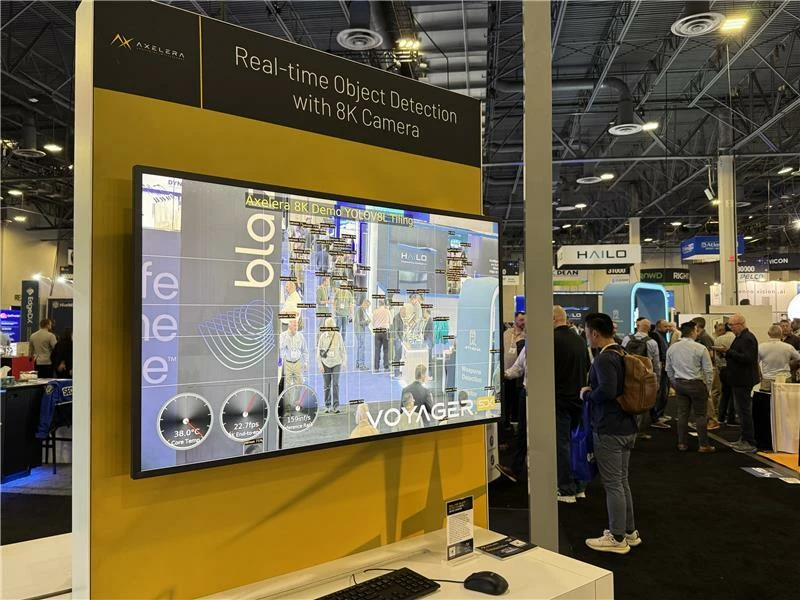
Real-World Deployment, Real-World Results
For the ISC West demo, we placed an 8K camera four meters above our booth - huge thank you to Axis who loaned us a Q1809-LE 8K bullet camera when ours got stuck with US Customs. From there, it could survey a section of the convention center floor, accurately reflecting how these cameras are actually being deployed in venues, stadiums, airports and more.
At this distance we found the optimal tile size to be 1280x1280, with each tile capturing a range of people and objects on the show floor. Using an Intel Core i9-based PC and two Metis cards, we were able to detect objects accurately with YOLOv8l at a rate of 23 frames/second. This equates to processing around 300 1280x1280 tiles per second. Moving to the smaller, but still very capable YOLOv8s model enables the same levels of performance using only a single Metis device, while our upcoming 4-chip PCIe card enables the highest levels of accuracy and performance.
The ability to easily change models and parameters using our Voyager SDK, simply by modifying YAML configuration files, makes it incredibly easy to build powerful systems for processing multiple high-definition camera streams at low latency and high frame rates.
It’s also testament to the flexibility that has come from building the Voyager SDK from the ground up to deliver ease of use and high performance at the same time, all within a single development environment. Building our SDK foundations to enable this degree of flexibility was not, however, such an easy problem to solve.
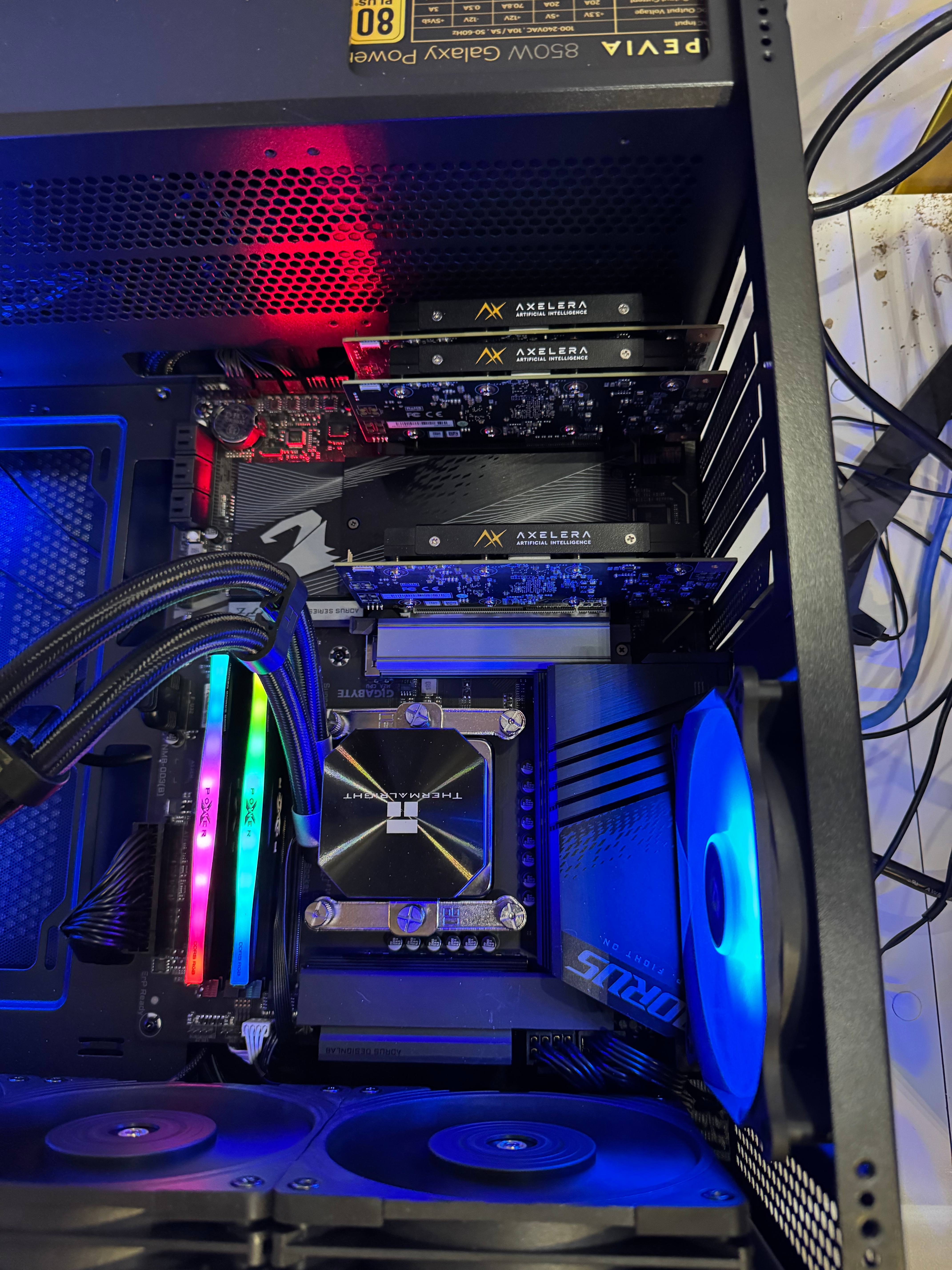
A Challenge in the Making
My journey with heterogeneous computing began a decade ago at GPU IP-supplier Imagination Technologies, where I worked with mobile OEMs trying to repurpose their GPUs for emerging compute workloads in the Android market.
At this time, the use of GPU computing in application processors was still in its infancy. Apple, as Imagination’s lead customer, was developing in-house features for the iPhone, and a fragmented Android ecosystem struggled to find compelling use cases in phones and tablets. Through various industry collaborations we did eventually achieve a few Android deployments, most notably (or perhaps notoriously) real-time camera “beautification.” But my feeling at that time was that embedded GPU computing was failing to reach its full potential, in part due to the difficulties of programming heterogeneous systems-on-chips using low-level APIs.
Fast forward to the last couple of years and we’re now in the midst of a major industry shift: the transitioning of compute from cloud data centers to edge devices, bringing end-user benefits such as reduced latency, enhanced privacy and lower costs. With AI playing an increasingly important role in products everywhere, many companies are looking to incorporate AI accelerators into their edge products.
Many of these products are already designed using host processors with embedded GPUs that offer impressive image processing capabilities. However, when it comes to integrating these capabilities within end-to-end AI pipelines, the state of the industry has unfortunately moved towards proprietary solutions.
Apple has orphaned OpenCL in favour of its Metal API, which, albeit very capable, is proprietary to Mac. NVIDIA’s ecosystem is firmly rooted in CUDA, also a proprietary API. At the same time, open APIs such as Khronos’s Vulkan has not yet delivered on its promise to evolve from a graphics-centric API to one that unifies compute-based kernels.
Against this backdrop, we set out to develop an SDK that makes it easy to integrate Metis AI accelerators with a wide range of host processors, while maximizing end-to-end performance by leveraging the image-acceleration APIs available on these hosts.
This challenge was just one part, albeit an important part, of the broader Axelera AI vision to make artificial intelligence accessible to everyone.
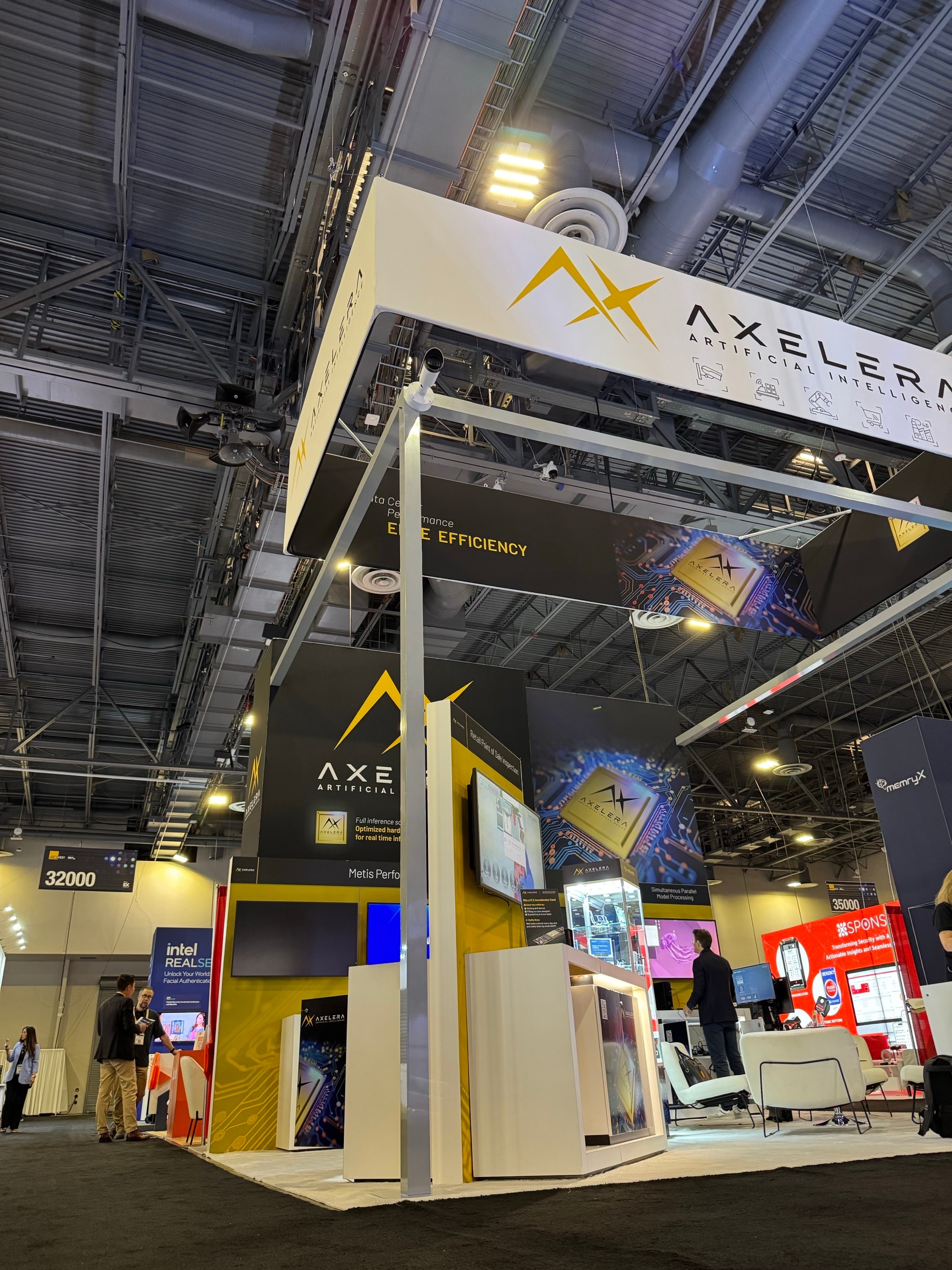
First Make it Easy to Use
We started by making it easy for developers to express their complete AI inferencing pipeline in a single YAML configuration file. Pipelines are described declaratively, including all preprocessing and post-processing elements, and optionally combining multiple models in parallel or sequence so that, for example, the output of a person detector is input to a secondary weapon detector.
We created YAML files for every model in our model zoo, using weights trained on default industry-standard datasets, and we made it easy to customize these models with pre-trained weights and datasets. We then developed a pipeline builder that automatically converts these YAML definitions into functionally-equivalent low-level implementations for a range of target platforms. We designed high-level Python and C/C++ application integration APIs that enable developers to dynamically configure these pipelines at runtime with mixtures of different video sources, formats and tile sizes.
At the application level, developers can simply iterate to obtain images and inference metadata, which can then be analyzed and visualized using Voyager application libraries. The Voyager SDK provides a single environment in which pipeline development and evaluation can proceed hand-in-hand with application development, from product ideation all the way through to production.
Then Optimize, Relentlessly
Working closely with early access customers, we prioritized the optimizations that mattered most to their use cases, like adding support for Intel Core hosts with VAAPI-accelerated graphics and Rockchip ARM platforms with OpenCL-accelerated Mali GPUs.
Integrating these compute APIs with other hardware, such as video decoders and the Metis PCIe driver, required careful consideration of various low-level issues, such as alignment requirements when allocating memory, and understanding which API interoperability extensions were supported most efficiently and reliably by the different hardware vendors. This was codified into our pipeline builder so that it can construct efficient zero-copy pipelines that pass only pointers between elements. Unnecessarily copying even a single buffer can substantially degrade performance on bandwidth-constrained devices, so a lot of time was spent considering optimal approaches for different combinations of tasks and devices.
With the core framework in place, we added optimization passes to the pipeline builder that fuse together different combinations of pre-processing tasks on the same device. This eliminates unnecessary generation of intermediate pipeline data that isn’t required by the application, saving additional memory bandwidth.
Over time the pipeline builder has matured into a product that can generate near-optimal implementations of many complex pipelines to meet the demands of real-world applications, and we’re excited to make it available to our broader community.
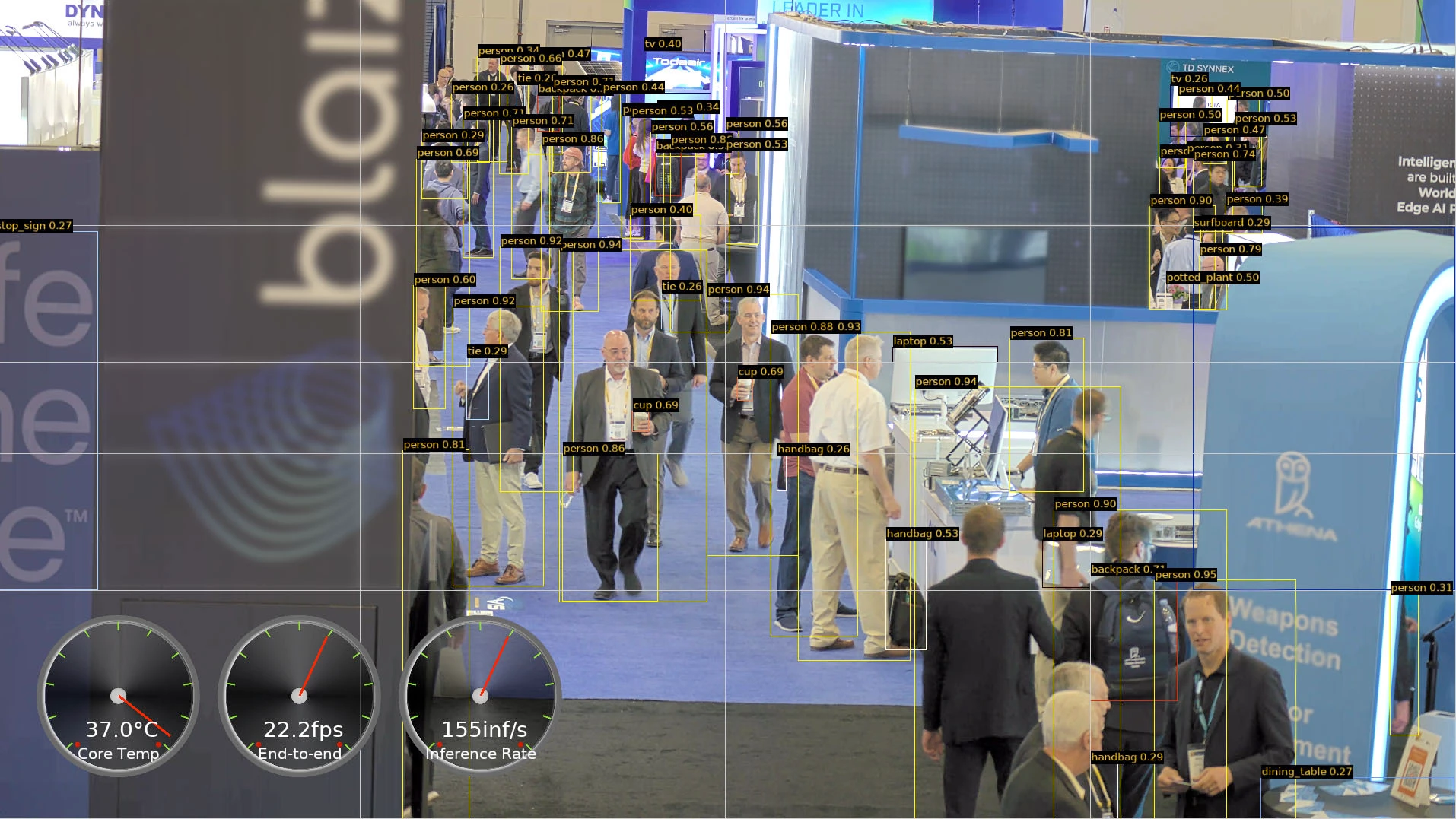
Where We’re Headed Next
The first public release of the Voyager SDK is a major milestone on a journey that offers many exciting opportunities.
As part of this first release we’ve also opened up lower-level APIs on which the pipeline builder and application integration APIs are built. These include a Khronos-inspired low-level AxRuntime API (available today in C/C++ and Python), which provides full control over all hardware resources used to build end-to-end pipelines.
There’s also a mid-level AxInferenceNet API (available today in C/C++ with Python to follow) that allows direct control of model execution from within an application (distinct from our highest-level API that fully abstracts pipelines to objects generating images and metadata). We’re excited to see how developers make use of these APIs, and how they would like to see them further improved. Feel free to share any such feature requests.
As developers continue to push the boundaries of what’s possible with AI, Axelera AI continues to innovate and ensure the broadest adoption of our products. For example, developers working with high-resolution cameras often need to manage large amounts of data within their application, from capturing and recording video in real-time, to scanning complex scenes over time to track, identify and analyze objects, and detecting key events in dynamic, real-world environments.
These are fundamentally difficult problems to solve, but by building tools that keep raising the abstraction level at which developers can create applications, I believe Axelera AI is perfectly positioned to deliver on the promise of making AI accessible to everyone.